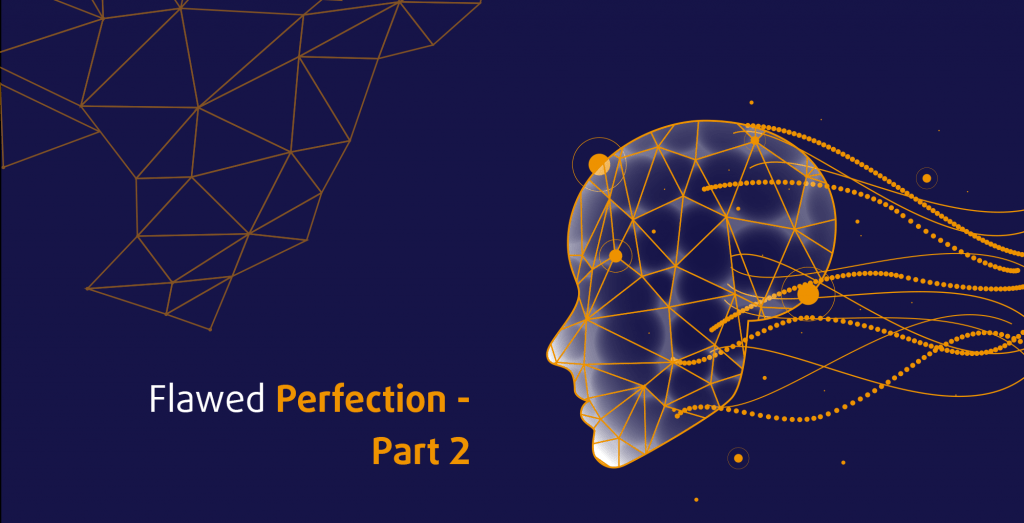
Bridging
In the first chapter of this article the construction to suffice with was used as an example to discuss the incompatibility of neural machine translation and informal language. Although the translations provided were not completely wrong, they could not convey the meaning of the original sentence. And when it comes to technical translation, nit-picking is the standard procedure. But I should probably get my own house in order before I dish out further criticism : neither is English my mother tongue nor was I aware of this specific use of to suffice with before I read the text in question. So I am no better than our test subjects, my personal data set also seems to be incomplete or contaminated. Nevertheless, I was able to understand the sentence as the author intended. Apparently, I (representing humans) possess an ability that the machine lacks at this point. How else would I be able to compensate for my own linguistic incapacity?
A simple answer would be something like: the context doesn’t allow any other interpretation! Or: humans have a brain, machines don’t. But since machine translation is a complex and fascinating subject, it merits an appropriate answer. So here is an attempt to explain this phenomenon on a linguistic-cognitive level .
It is true that the meaning of the sentence is made clear by its context. In this case by the second main clause of the sentence, because unlike its predecessor, there is only one way to interpret the aforementioned dependency. Even our test subjects agree on that. This transfer of information can be explained in theory by an indirect anaphoric connection, also called bridging. In short, an anaphora is the reference in a clause to something or someone previously mentioned.
A good example of this is a personal pronoun, which establishes an anaphoric connection to a person or a thing introduced earlier in a text. An indirect anaphora is a bit more abstract, because it is not based on grammatical rules but rather on world knowledge. A simple example shows how a string defines a later one through bridging:
Brexit vote in the House of Commons: Serenity in Brussels
The word “Brexit” activates the part of our world knowledge around Britain’s withdrawal from the EU. Based on that, we later interpret the word “Brussels” within that familiar framework. The exact same framework-based interpretation will most likely affect your perception of the two following, legitimate paraphrases of the same sentence:
Brexit vote in the House of Commons: EU Parliament does not panic
Brexit vote in the House of Commons: The relaxed inhabitants of Belgium’s capital
Bridging helps us to choose the correct translation or paraphrase. For the same reason we are able to understand our original example: due to the use of a construction unknown to me, I’m unable to determine the relation between machine-to-machine application and SIM cards. However, since the same relation is unmistakably defined in the second part of the sentence, my world knowledge links the two examples, thus making sense of the first part retrospectively. And as if that wasn’t enough, I also improved my own algorithm by learning the alternative use of to suffice with, independently and automatically.
NMT models can certainly handle context, e.g. by including specific TMs or high-quality language corpora. That said, a complex contextualization is still a dream of the future because it requires artificial intelligence that is on par with that of a human when it comes to cognition and recursion.
An outlook
Informal language and the inability to properly process context add up to a long list of unaddressed tasks in the development of human-like NMT engines. However, you would have to be very naive to deny the capabilities of neural machine translation. No matter how abstract and complex these examples might be – I personally have no doubt that one day, these problems will be overcome by the machine. Despite my confidence in NMT, I wouldn’t dare to guess when that will be. Until then, only a small number of translations will be fully automated. That prediction also takes into account revision and maintenance of NMT engines. For the time being, humans still have the final say when it comes to translation, not to mention localization. If you are still looking for the right humans for your translations or localization projects, we at text&form will be happy to help you find them.
About the Author